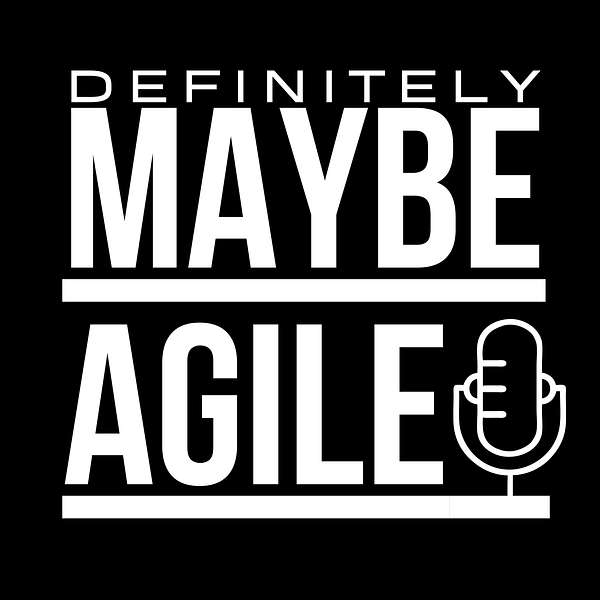
Definitely, Maybe Agile
Definitely, Maybe Agile
Beyond Big Data to Smart Governance
In this thought-provoking episode of the Definitely, Maybe Agile podcast, Peter Maddison and David Sharrock dive deep into the evolving world of enterprise data management and its impact on modern organizations. From data hygiene to governance, discover how the definition of corporate data has dramatically shifted in recent years and what it means for your business.
This week´s takeaways:
- How regulatory compliance is reshaping data management practices
- The critical balance between quantitative and qualitative data in decision-making
- The impact of AI and LLMs on data analysis and sentiment tracking
Whether you're a technology leader, data professional, or business executive, this episode provides insights into navigating the complex intersection of data governance, team structure, and business value. Learn why traditional approaches to data management may be insufficient for today's challenges and what successful organizations are doing differently.
Welcome to Definitely Maybe Agile, the podcast where Peter Maddison and David Sharrock discuss the complexities of adopting new ways of working at scale. Hello Dave, how are you today?
Dave:Peter, great to see you. I feel like your accent should have got stronger after the last couple of weeks.
Peter:Yes, yes, it might have got a little croakier because I feel like I've been talking all day, but you enjoyed your trip to the UK. I did, I did. It was fun over there and it's always good to go home, always that home. I think this is home, yeah for sure.
Dave:For sure. What are we talking about today?
Peter:We're going to talk about data and how data has evolved over the last several years and how that's impacting the teams and organizations and what all that might mean.
Dave:Yeah, and I think, to be fair, this is going to be the first of several conversations. It just feels, even as we're trying to get some structure to it, there's a lot to discuss around this topic.
Peter:Yes, yeah, and it took us a while to even get to the point of narrowing it down. So what do we want to talk about? And I think that it's an interesting point because I always, as I was describing to you before, I think of it as like it's the multiverse of data. We always talk about the coding layer and everyone always describes the coding layer and there's this data space over here, but they're kind of these dark wizards and they're not really as included in the conversation, despite being essential to all of it, and how that intersection occurs is sometimes not always done in the most effective way.
Dave:Well, I think the other thing is it's becoming a topic at the executive leadership table. I mean, it's always been there as part of some sort of regulatory perspective table. I mean it's always been there as part of the, you know, some sort of regulatory perspective, and it's always been there in the sense of we want to look at some information about how the business is running or where this is happening and that's happening and so on. But it's now a topic in terms of just simple things like you know, what data is ours? What do we own in terms of the data? Are we using it in an appropriate manner? Are we collecting it and managing it and doing all of those sort of? I almost think of that as the hygiene side of things. That was less critical is now top of mind in many, many organizations.
Peter:Yeah, I would say that the conversation has changed. It's gone from hey, data's the new oil. We've got to have our data. Let's put all our data together in one place, let's spend all the money in building these data lakes and then we'll be able to get all these wonderful insights and we can do all this analysis against it and maybe we'll discover some new business models out of all of that. It's shifted a little into the one you were describing more, which is like data is a critical conversation. It's critical to everything that do. What might we do with the data? Which data is ours? How do we make sure it's the right data? How do we understand if there are biases within that data or if we're making the wrong decisions as a consequence of data?
Dave:But I feel I agree with what you're saying about some of the ways of using the data, but I also I agree with what you're saying about some of the ways of using the data, but I also feel that there's a there's a stage before that, which is the stewardship of data, and this is a little classic situation of the regulatory bodies are now catching up with the push that has been the last decade or so around big data and around data driven decision making, and and there's been a sort been a lot of data scraped, if you like, pulled together Scraped is probably the wrong phrase, but pulled together over years.
Dave:And now, of course, the regulations are coming in and they have teeth, and now we're looking at those to say what data are we collecting? What data have we collected and are we managing? Are we stewards of that data in the right way? So there's that element of it that comes in and obviously raises a whole bunch of things. But then the second piece is how are we using that data? What decisions are we making? Are those decisions being correctly informed? Are we slightly better than we would be without the data? Are we making poor decisions? Biases, whatever else may be in there, like data integrity, which is not there, so that we're making assumptions.
Peter:The role of the CDO, the chief data officer and this type of role coming into organizations, but doesn't seem to last very long.
Dave:Well, and I think this, is a natural and we've talked about this many times which is, when you stress an organization about something specific, the natural way of responding to it that we've been taught to do ever since we've been in school and university and so on, which is to impose a bunch of rules onto the system and constrain that system.
Dave:So, whether it's any time you now build an application, you have to create some form of data integrity audit before you can move forward. Or you now have to get approval from certain CDO, basically the CDO or their team, so the first response is always put a whole bunch of constraints on the system just to kind of somehow manage it.
Dave:It has to be done, or at least it's a step that many organizations are not going to be able to avoid. But it's actually a mindset. It's a cultural change of how we think about data. So now that's not going to change how we think about data. It's actually a mindset. It's a cultural change of how we think about data. So now that's not going to change how we think about data. It's going to make us get frustrated about working with data. How do we actually move that organization into one where data is one of the levers that they're thinking about, that they're understanding and working with and using in a correct format?
Peter:Well, this is one of those pieces of we, as with a lot of other things, there's an education component of what do these different things mean? What do these terms mean? There's a regulatory protection piece. There's like is this personal, identifiable information? Is this things that I need to make sure of under lock and key, that are not easily accessible? But a lot of this then has to come back to from the person who's building the systems or is incorporating that data into things that they are doing. Are they aware of what data they should have access to and shouldn't have access to? Are they appropriately working with that data? Is it being managed correctly? So, I mean, which is where you end up with one of the organizations who have a lot of this type of data will put strong safeguards in place, especially around very sensitive data, to ensure that that sensitive data does not end up in the wrong places.
Dave:As you're saying that, I was even thinking that if we had this conversation a few years ago, the things that we would, that terminology, that term itself, has drastically changed, even in the last couple of years, because nowadays, if you think about things like the images that an organization has created, or the information that they're getting through, say, the recruitment, hr or through customer service and things like this.
Dave:All of that could now be considered data in the terms of it now has value in terms of how that might turn the business into a better business, and it's not something that would have been talked about, maybe even five years ago, as being, you know, under that umbrella of data.
Dave:So, even as that, data constraints are correctly becoming tighter and the need to protect sensitive data, to re-influence that, to have very clear accessibility, rules and governance and these other aspects which are coming together, the understanding of what data is has broadened to the point of it's very close to saying anything we have on our servers.
Peter:Yes, yeah, I see your point there that it has, because, I mean, we've always tracked these, they've always existed in sort of repositories, they've always been there, but now it all comes under that same purview of needing the same sets of protections, and so this is also something that we need to ensure is managed in a similar fashion, are we? Because it potentially impacts other types of data, because we can now have the ability to draw these things together and start to build understanding across disparate pieces of information like this, whereas we're truly getting to information technology at last.
Dave:There's already a distinction between data and information that I'm not proposing we explore right now, but yes, you're absolutely right.
Peter:Yes, and that's a good point. Yes, All information knowledge, data information, knowledge, and then yeah, but maybe so.
Dave:Having just started, this conversation tugged on a few threads and now it feels like we're now dealing with. The universe is expanding at an increasing rate. Where are we going to?
Peter:go.
Dave:What is your observations? What are you seeing with the teams and the organizations you're working with as to how they're tackling this?
Peter:So well. There's a couple of things that are coming down. One is that I mean there's a greater focus on it. There's a focus on what is our overall strategy around this. How are we going to ensure that the various controls we have are in place? How do we do that without impacting our ability to deliver? And so teams looking at this from the perspective of how do I make sure that the right parts of data are properly protected.
Peter:So you're seeing a lot more understanding around data categorization. So seeing that become sort of concept of data cataloging and data categorization and associating that to particular types of data has been something that I've been seeing more of, because that then gives you the ability to say which data is what type, certain aspects of well as I was mentioning before, that kind of like on the business side, the CDO office coming into existence and attempting to provide some business level guidance as to what needs to happen with data. Seeing that as well, I do see at the team levels, there's still I've seen in some organizations quite a bit of fracturing between the different roles, because there are so many different roles in data and getting them to collaborate across those different roles can be something that is quite tricky between the people engineering the data pipelines to the people managing setup of the data analytics tools to the people who are doing the analytics, and getting alignment across these different groups to have them function well and cohesively is something of a challenge.
Dave:Yeah, so if I just summarize, there's a couple of things that I'm hearing you talk about there, peter. One of them is I always think of it as data hygiene, but that's or actually the model that was in my head is I've had several experiences, having lived in lots of different places, but several experiences of cleaning out the attic or cleaning out the garage or cleaning out the basement.
Peter:And it feels like a lot of organizations are doing that around the data.
Dave:They've got boxes of data that they've gathered because back in the day, organizations in many cases were gathering a lot more information, a lot more data than perhaps they needed to, a lot more data than perhaps they needed to. So they're now having to kind of sort that out, categorize it, make sure it's properly managed, that sensitive data is separated out, that they've anonymized data sets if they're using them for various different purposes, and so on.
Dave:So there's that side of things is almost like the cleaning up. I think of that as cleaning out the attic type of thing, organizing things, something that all organizations should be or well on the way to doing, if they're not completed already in many cases. And then the other side is how we're working with the data, and I'm continuously reminded of that phrase in Lean, which is optimize the whole. And one of the headaches with data is you're very often it's unclear who the customer is. If I'm working with data, it's unclear at what point I'm handing off to the final customer or end user of that data, because I'm often handing off to I have no idea a business analysis team which is at least one step removed from the decision makers on the executive team that are using that data and the models and the analysis that comes from it to make decisions. So there seems to be a gap where, if I'm building a web application, I can see who is using that. I know the user much more closely than I do if I'm working in data.
Peter:In many cases, yes, you're a customer quite often. Well, if you're doing the data pipelines, your customer in data is going to be the business analyst very often, or the analyst who's doing the creating of the reports, or they're doing the analysis of the data. Versus if you're the analyst, of course you've got more access to the customer, presumably because the customer is the person who's taking your analysis, so that you can get some feedback in that fashion.
Dave:So what I struggle with that, though, is there are decisions being made that aren't followed all the way through. Yes, so one of the continuous conversations that we end up having working with data teams is trying to get the problem to be solved passed down, rather than the data requirement to be passed down. If you get a requirement that says I need the address, it's for all of our customers in this state. It's very different to understanding what the problem is that we're trying to solve, which getting the addresses for people customers in a particular province or state is going to help us solve, and that kind of gap is very wide. It's very rare that you come across any team that knows the problem they're trying to solve, apart from a generally make the business better.
Peter:Yeah, and I mean, it's not the only area and it's a problem that's existed for a very, very long time. But it is true, yes, you end up at the back end. You're asked to put things together without necessarily understanding what is the business problem we need to solve, and if you were made aware of what the business problem you need to solve is, then you could probably come up with a different solution for it or provide an alternative that might be smaller, shorter, faster, get you to market faster yeah, but that requires transparency of what the business problem is across every layer of that stack, and that quite often doesn't happen but.
Dave:But I also think there's an urgency around that, because now a lot of this data is being used to actually make kind of real world decisions in real time. So if we don't really understand how that data is going to be used and and to solve it, there's a very real risk that we, that we're kind of teeing things up for a bit of a crunch, a failure of some sort.
Peter:Yeah, so I think there it's a. I think the point that you're drawing on there is that, yes, when setting up data teams where data is going to be a core part of the application, where you're serving up something to a customer make sure that your backend data teams are the ones who are either collating or generating or bringing data in are a part of the conversation so they can understand the problem you're trying to solve and don't keep it off to one side and send them over with service tickets all the time. Make sure they're a part of the solution so they can understand how to help you solve it.
Dave:Now, what about things like? So? One of the other things that I often see is quantitative data carries much more weight than qualitative data. Sense making is deprecated in the sort of world that we live in now, where, if I can you know, statistically analyze a whole bunch of data and come up with some sort of probabilistic models. I'm going to take that over, sensing anecdotal and qualitative information alongside it.
Peter:Well, I think that's always been the case, but I think that actually LLMs this is actually one of the things that they are bringing to the table is that you've now got much more effective and much more efficient and I use that somewhat dubiously, given the power consumptions that we see in the news these days but the LLMs do provide a much easier path to getting sentiment analysis from data, providing you are able to feed whatever you're collecting into an LLM to get that, and there's various ways to do that inside of the firewalls if you need to. But that ability to capture that sentiment analysis and turn that into something that you can then more easily quantify essentially is what makes it easier to consume.
Dave:I agree that there's an element of that coming in. I'd still say sensemaking has such a deeper value to it and it's agreed it's never really been a peer of quantitative analysis. But in a world where quantitative analysis is dominating and has teams being built up around it, I think the sentiment analysis is a tiny tiny. It's scratching the surface of what can be done around that and it's still something that is missing in many of the analyses that certainly we're part of and involved with.
Peter:Yeah, I would agree, and it is absolutely critical, because if you're not actually listening to what your customers are saying, you may very well miss things, and I think we've talked about that in the past too that you might be getting X number of transactions, but if you're not listening to your customers, you might find they're all about to quit. There's a terrible example.
Dave:Well, and then we talked about this with the Nike story a few podcasts ago, and that was a great example. Well, and then we talked about this with the Nike story a few podcasts ago, and that was a great example of quantitative data missing the mark and qualitative data really being the critical element in that story. How do we bring this to a close, or at least a pause, because, as I said, I think there's plenty for us to discuss here?
Peter:Well, I think we've covered some interesting topics. I think there's plenty for us to discuss here data hygiene and the criticality of that, and although data has always been and it's been evolving a lot over the last several years, of course, and as a part of that, we're seeing more of a focus on, hey, how can we not only get the kitchen clean, but how can we keep it clean on an ongoing basis using different cataloging mechanisms and association metadata to different types of data, and so we can start to solve some of these metadata to different types of data, and so we can start to solve some of these problems. Potentially with some of those and I think actually some of the other pieces, like LLM type technology, other bits can help you with some of that analysis. But anyway, what would you?
Dave:add to that. I was just going to say I always love these conversations, peter, because you pick up a completely different strain of the conversation to what I'm picking up. So what I was picking up is, first and foremost, is that cleaning out of the attic and just getting regulations of quarter, there's a whole bunch of work being done in terms of categorization and clarity, making sure we're gathering the right data not tons of data how we're using it and all of the things that go with that. Totally agree with that. Totally agree around that. I think.
Dave:The other thing that really sprung to mind, or kind of stuck in my mind as we're having this conversation, is the definition of data has changed, and I think that's both an opportunity but also incredibly problematic, because many of the frameworks, tools, structures that we have in place to deal with data are dealing with old school data, not the new definition of data. So there's going to be plenty of room for misunderstanding, miscommunication, believing something has been taken care of when actually it hasn't, for all of that different sort of types of data.
Dave:And also of course, that also means there's opportunity there for understanding how all of that data can be valuable to the organization, which we've not even touched on. We'll talk, I'm sure, another time. And then I think the other bit is that and I keep coming back to the sensemaking side but there's a huge imbalance between quantitative data, data that I can put in a table and see, and other forms of data, and we talked about it with the Nike story a few podcasts back, but it's I think there's more and more anecdotes coming out about this sort of misunderstandings that can happen because we only rely on quantitative data Again, topic that we've scratched the surface on many times. Yeah, I'd agree with that.
Peter:Cool Well, thank you, dave. It's always a pleasure, until next time.
Dave:Always a good fun, always a good chat, isn't it, peter? So good, welcome back to the Canadian shores and look forward to the next conversation, you too.
Peter:You've been listening to Definitely Maybe Agile, the podcast where your hosts Peter. You've been listening to Definitely Maybe Agile, the podcast where your hosts Peter Maddison and David Sharrock focus on the art and science of digital agile and DevOps at scale.