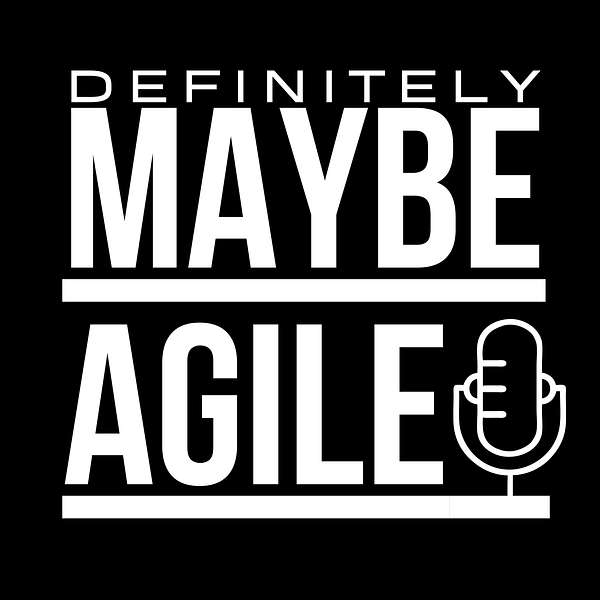
Definitely, Maybe Agile
Definitely, Maybe Agile
How AI Agents Are Transforming Enterprise Data Work with Suzanne El-Moursi
In this insightful conversation with Suzanne El-Moursi, co-founder and CEO of BrightHive, Peter and Dave explore how organizations are addressing the growing gap between data volume and analytical capacity. Suzanne reveals that while 90% of the world's data was created in just the last two years, only about 3% of enterprise employees are data professionals, creating a massive bottleneck where business teams must wait in line for insights from central data teams.
BrightHive's solution is an "agentic data team in a box" – seven AI agents that work in unison to handle the entire data lifecycle from ingestion to governance to analytics. Unlike typical AI solutions, these agents operate at the metadata layer to ensure quality, compliance, and meaningful insights without replacing human expertise.
The conversation covers compelling use cases across industries – from helping resource-constrained organizations extend their analytical capacity to unifying fragmented data landscapes resulting from mergers and acquisitions. Perhaps most striking is Suzanne's vision for measuring AI's impact through what she calls the "delight KPI" – are employees finding their work more fulfilling when augmented by these tools?
Key Takeaways:
- Data fragmentation persists - Organizations struggle with siloed data across systems, especially after mergers, blocking comprehensive analysis.
- AI augments human intelligence - "A doctor with AI will displace a doctor without AI" - the goal is removing grunt work so humans tackle higher-value analysis.
- Measure the "delight KPI" - Track how AI improves job satisfaction by enabling more data-informed work without technical bottlenecks.
- Cultural shift needs technical solutions AND organizational buy-in to overcome skepticism about AI in the workplace.
Welcome to Definitely Maybe Agile, the podcast where Peter Maddison and David Sharrock discuss the complexities of adopting new ways of working at scale. Hello and welcome. It's wonderful to see everybody here today again, and I'm here with Dave and Suzanne, so it's a pleasure to have everybody. And so, suzanne, would you like to give a brief introduction to yourself?
Suzanne El-Moursi:Absolutely. Hello everyone, my name is . I'm the co-founder and CEO of BrightHive. Brighthive is an agentic platform for the enterprise data work. We are accelerating and excited to accelerate all of the different mountains and valleys and hills of data that we have in the world. We're excited by the fact that 90% of the world's data was produced in the last two years and our platform mimics a fully end-to-end data team, when you think data scientists, data engineers, all the different nuanced subspecializations of data engineering as a whole into seven agents, or seven agentic AI agents or data agents that are not a wrapper on any singular LLM and they work in unison to give enterprise work, staff, employees, teams you know, just people, knowledge, workers, a full data team in a box to help them break down and process from data to insights.
Suzanne El-Moursi:That's us. We're a startup out of downtown Chicago. We've been around since 2018 and have always been obsessed with the data problem. Working with data is hard and it's time consuming, so wouldn't say that we're just. You know we were always a data company, so wouldn't say that we're just. You know we were always a data company, but we believed in the power of AI and we're already working on that kind of in the sandbox before ChatGPT showed up on our phones in November 2022. And it was really a stroke of luck for BrightEye at the time because then we had conviction already around the potential of AI and specifically agentic, and that's when we rolled it out and became even more front and center in the marketplace for enterprise work.
Dave:So, suzanne, that sounds like that's an acceleration moment. You're literally like all the afterburners are on at that point because there's a lot of attention to it and new capability that came out as a result, or at least enhanced capabilities to what you were building.
Suzanne El-Moursi:You hit it perfectly, dave Absolute acceleration moment, a struck of luck, whatever you want to call it and we're meeting the moment head on. As I mentioned, in 2018, our obsession with data work is hard. Just the on-ramp of working with data is hard. We all know it. We've worked in all different kinds of organizations and varying sizes where you know that the answers and the data assets that you know the company has or that you have, whether you're in strategy, operations, finance, also cross-functional you know that it's fragmented and siloed. You know it's hard to extract it. You often wonder on its cleanliness and usability, right, and what that has created over time is actually a complete bottleneck and a difficult on-ramp right for everybody in the company. Now, the other piece of data that we found is, on average, the median percentage of data professionals in the enterprise hovers around 3%. Professionals in the enterprise hovers around 3%, and the volume of data and data work that we have is multiples of 100x. So really, the data engineer to data work ratio is completely whack right, and what we know is that business teams across any organization McDonald's to Coca-Cola to whoever rely on these central data teams of limited capacity data engineers and such so that they can actually get them to help unlock the insights in these data assets that span the enterprise. So when we did basically our research, we found, yes, business teams are frustrated. They kind of take a number and stand in the queue and wait to essentially be picked up by the central data analysis teams. Very rarely do you find organizations actually have data engineers in every function, right, they're usually central and so therefore you've got a choking kind of situation where a lot of people, a lot of demand, very limited supply. And when we looked at, okay, this is interesting, there's an opportunity in that. So you know, we'll always have been trying to solve the very ugly problem, right, of accessing data in a more fluid model.
Suzanne El-Moursi:We also found that when we step back, the entire marketplace of data tools. You know, the modern data stack is like a double-edged sword. As I explain it, it's terrific in the number of innovations we have. We have phenomenal brands, right Lake houses, warehouses, data tools, transformation, etl we know all of them and therefore you have innovation on very specific things and very specific tasks. But when you step back and put yourself from the non-technical point of view, it's a crowded marketplace, right, there's too much choice and you want to invest in your data infrastructure as a business leader and as an IT leader, but, again, too much choice. So when we looked at the marketplace, if you take the cost of tools alone, which is between three to 500K a year half a million at least and that's a medium sized organization, right and then you add the salaries of those data professionals, you're talking on the low end somewhere between 1.2 to 1.3 million dollars for about a team of four people and the tools that they need to much higher than that as bigger, you know, as the organization gets big. And so we kind of also saw, well, a lot of the organizations in the world and if you just look at the United States alone, there's between 500 to 5,000 employees, that's 35,000 registered entities in that segment, and that's a $4.6 trillion town. Those folks don't have a modern data stack, haven't invested continually in their data infrastructure, right, but they would like to do that.
Suzanne El-Moursi:So as we began to define the bright, high value proposition, it centered around these two things that we want to essentially make data work. All work become data informed work, right, meaning the work that's happening in the business units, in the business teams. How do we relieve kind of this bottleneck of access to trained professionals. You know you question a dashboard. You want to look at the field in the warehouse. As a business user, you don't have the tools and the knowledge to actually go into that infrastructure. So how do we open up and allow everyone to have that access to do the type of data analysis that informs their work and make it data-informed work? And just think how powerful that becomes when anything you're proposing to your leadership or any task you want to kind of question or any next step you want to define is evidence in the data that you have and you can point to answers and you can point to that.
Suzanne El-Moursi:But then, secondly, we found that there's quite a bit of a market for the SMBs where, with one buy, they're getting a full data stack from BrightHive, because our managed data stack gives you a data infrastructure. We need that so that our agents are very powerful. Right, and, of course, if you don't need that, you will be in our different deployment where you're taking our agentic layer on your stack. You don't need us to help you with Snowflake, for example, and there that in itself made us composable. We were already solving the infrastructure piece. We were already solving the data collaboration and sharing piece. When promise of AI became evident to society, that's when we knew our agents that were in the sandbox. We pushed them out fairly quickly between mid last year to where we are today. Literally now it would be a year right. So then, how do we create agents that mimic data tasks when, also, you're going to tool all the infrastructure that comes in?
Dave:So both Peter and I are listening. This is an area we both of us have a lot of interest in, so I think we're kind of just like absorbing everything that you're sharing. But what I wanted to do is just bring in a little bit around the teams. So, again, we often talk about well, we're seeing in the marketplace a lot of conversations about how AI is coming after this. You know, in this sort of the sloganeering of coming after certain jobs and so on, in the sort of the sloganeering of coming after certain jobs and so on, Data is this really interesting space because it's so technically driven that there's an obvious, like really great opposition there.
Dave:Can you maybe talk about a use case where the agents that you're using or you're offering work alongside existing data teams and how that might work and how they're? I mean, is this those teams training their replacements, or are they really empowering, you know, enabling those teams to be able to get through a bigger backlog of requests? What are you seeing from the use cases that you're seeing when the agents are sitting alongside existing teams?
Suzanne El-Moursi:Without sharing customer names. I'll tell you their use cases. I'll tell you two separate things. So, first of all, when we're being considered, we're a CIO, cto, cdao, buy so we're on the technical side of the house, so we are vetted very quickly, early on, as a tool that is going to be powerful. First of all, and how do you even do what you're saying? And when we try to figure out so great, we vet, check the box. So what are you trying to get out of us?
Suzanne El-Moursi:And there's consistently two scenarios that they're asking and wondering if we can solve. For Number one, I'm a CIO with one data analyst and the organization needs a lot of insights, not so much the data engineering, but just needs to refresh the dashboards. And it's exhausting right when we haven't been able to find the data analyst or we have them start and then they leave. So in this case, we want to have a self-servicing and in fact, I even am stealing this from my client because he said that. He said we're growing as an organization and I lead the data team and we support so many dashboards that, across the business, from customer success, from marketing, procurement this is a wearable in the health care category Great, and the reality of it is, I can't get enough people just to be able to help create the data pipelines, because you need the data pipelines and transformations so that these dashboards serve the business and at this point, I'm not really sure we need dashboards at all. So is there a way for us to say, this dashboard as of like last Friday, but with this tool, which is mimicking the skills that my data analysts have? With this tool, you can actually go to the source at any hour. You don't have to wait until a dashboard is updated or you don't have to wait until we actually make a push. And the immediacy of the insight allows majority of the case studies that there are use cases that are being asked to support actually be self-serving within the organization, relieving the load off of his analysts to do a whole lot of different planning and future scenarios, because it's in a growth stage, okay, and this is a proudly held company.
Suzanne El-Moursi:And so to us, we're like okay, 80% of this work is just grunt data work. Right, those engineers that you take a while to find and let's remember that data scientists and data engineers in general and data analysts are hard to find and are expensive. So the value proposition you're increasing headcount without increasing OPEX. You're removing the grunt work off of the few analysts that you have. You're utilizing them to meet the kind of the strategic agenda of growth planning and scenarios that are about the future of the business for growth into a five year out plan. But you're also not abandoning your responsibility to keep your business team completely plugged into the insights that they need, right, and when you're in the consumer space, as you know anything consumer packaged goods or consumer electronics you are looking at things literally on a weekly and a monthly basis. A quarter is too far away, right, and so that scenario of how does my limited team meet both the work that's necessary for future growth and business needs today Definitely not training anybody to take anyone's jobs You're almost delegating 80% of the grunt work to almost, you know, offshoring those.
Suzanne El-Moursi:I mean, we did this, we offshored in the last decade to all the different places in the world, except now they're right here. You can monitor you know, monitor the security of the agents, you can monitor the performance of the agent and you can keep much more tab, a closer tab. The other scenario is actually very unique and we're finding it pop up even a little bit more more commonly than we realized, which is organizations that are large already but through M&A. So think about all the data that comes from M&A and where does it live. So there's quite a bit of companies that just go through that and now you have such fragmentation to essentially get and usually they're PE backed or PE purchased or became private from a public space. They have a five-year trajectory and they need to be flipped private from a public space. They have a five-year trajectory and they need to be flipped, and the very first year is just figuring out all of the M&A that has happened.
Suzanne El-Moursi:The most important asset is the data to be able to find all these synergies. They're not having enough people process all of that data, right? There's some stuff still on Tableau desktop for one of these clients, customers that we're working with, right. So the aggregation, not even to get to the analytics level, but just to be able to do the pipelines and the infrastructure and with it they're considering where can we have cost optimizations on the tools? Because when you're purchasing from multiple places, you're acquiring the data in the environments that it's in, and so when you look at the streamlined efficiencies, you need to do in your infrastructure alone so that you can move the rest of the data lifecycle. It comes down to OK, we want the agentic layer, but then also, what other bills can we sunset, like a Fivetran bill or whichever one, because we're getting it part of the buy. So it's basically people and resources almost always.
Dave:So, and what I'm hearing you say there is that this is really an acceleration tool or, you know, allowing the existing data engineers, data scientists, data analysts that an organization will have, but it's effectively giving them more power because the routine or the grunt work gets taken care of Out of the box. Like thinking about the problems and crafting those problems in the right way.
Dave:What about interaction with, so one of the things that we recognize a lot of the time, so we talk all the time about and, peter, you're going to have to jump in at some point- otherwise I'm listening.
Peter:I mean, I do have some questions, but you're on a roll, so I'll let you go on.
Dave:Let me ask one more question, then Peter's going to get a go, but one of the things that we often see in an organization, we talk about getting value out of the door. I mean, from an Agile DevOps perspective, it's very much about a learning cycle that allows us to deliver something, learn from it, bring it back and so on, and in a business world, often they don't know how all of that data is there.
Dave:It's got tons of information but they don't know how necessarily to ask the questions so that they get something coming out Again. Any use cases around that or what's your experience?
Suzanne El-Moursi:Oh, absolutely so now. So first of all, let me just explain the agents themselves. So there are seven agents. They're mimicking the phases of the data lifecycle. So now we're talking about quality data work.
Suzanne El-Moursi:It doesn't matter what your title is as a person. This has to happen, like governance. You must govern your data, especially if it's high PII. Agent essentially guides the user to identify wherever PII may be, flashes it for the user, walks you through basically a workflow of do you want to restrict this? Like, for example, social security and birth date? Never together, right?
Suzanne El-Moursi:So that's what we mean when we say we are agentifying the mission-critical tasks that have to happen to make sure that this is quality data work. And we're working at the metadata layer. We're doing that faster because we're giving you an agent that has been designed and engineered to do that, right. The business user doesn't know anything about governance, right? And so, basically, that guidance with still a human in the loop to get you through being able to govern the data. You could also be a technical person, again, that's a delight, because then it's click and you're fine and it's done it for you, and then the same thing you know when you think about one step before that the ingestion agent to be able to look at all the air bytes and the different sources flat files, structured data, pdfs, unstructured, like all of it comes into a central place and essentially clean and create a schema and glossary all of that part of it as well, right Before you even get to the prompt.
Suzanne El-Moursi:Why am I highlighting this? Well, the agents, they're artificial until they're not, until you teach them. And so guess what we get to brag about is that not all AI is equal. And our AI is pretty damn good because we clean and create all the plumbing for data pipelines to teach these agents that then help you answer the question so you could enter a prompt that isn't necessarily the right way to ask the question, and so our agents are going to essentially respond back and become more insightful in the response, because they're telling you perhaps you should be looking at X, which seems to have a better distribution of relevancy to your question and gives you a distribution with a visualization.
Suzanne El-Moursi:So when you start with garbage and data, you don't get great outputs, right.
Suzanne El-Moursi:So it starts from the data itself, the pipelines that make the agents more intelligent about the context, right, All your data is about context. We create that in our RAG, and then the engagement for you as the user, basically a graphical user interface, that is, a agentic prompt language, and that's where we're investing more with the help of our agents themselves how to write a prompt, how specific let's actually call the column, the header of the column in table X, and be very specific in that. Business users would have never had even the idea to do that, right, but guided by an agent with a human in the loop, it is both refining your prompt questioning and relieving much more insightful answers than the obvious answers. Right, a VP of sales should know what was the biggest sale for them that quarter. That's not insightful, right? But how does it actually match up for the last 10 quarters and on what parameters and what price differentiation? You know what kind of discount had happened or hasn't happened. That becomes something possible because the agent can bring that forward and show it.
Peter:I liked one of the pieces you touched on there around the compliance aspect of it, so the ability to ensure that the compliance step gets done correctly. And we worked in a lot of very heavily regulated organizations, a lot of them in financial services, and one of the big challenges we run into there is access to production data to do the data analytics in the first place, where restrictions mean we can't access that data. But not being able to access that data means the analysis is largely meaningless because you can't have it both ways right. So have you run into that or have you and your agency, your company, helped deal with that type of sort of quandary in the past?
Suzanne El-Moursi:Yeah, well, so the fact that we've baked governance into the platform in our workflow, that it's not an afterthought. So, for example, we wouldn't be pulling any data that doesn't have a governance layer on it, because it is part of the steps of the workflow. You can't touch it in the old world because we don't know if governance has happened in the first place. So the scenario you're painting you just can't go, grab data off the shelf or from the warehouse and be able to say I'm going to use that. Compliance is going to be all over you. Who are you? Why are you touching this data? It's restricted on all of the pieces.
Suzanne El-Moursi:So all these checks were happening by human beings, but they weren't necessarily controlled and policed by any specific software solution. Often, or if they are in really big banks too big, too fragmented they cannot confirm it from one function to the next. Today, as our clients are, by the way, none of their data comes to us. They're applying our agentic layer on their cloud or they're doing the managed data stack. So we are able to show, because we have the governance agent, that that actually has happened and here's what we're extracting. Also, we work off of the metadata right and when they want to take the raw data in, they get to see that none of the data is on our cloud or in our hands, right?
Suzanne El-Moursi:So we really separate the church and state and we show that we've identified the most important steps, which is making sure that governance was applied, and then the system is able to show. And then just think about being within the same, different functions within the same company, or being multiple organizations as data partners. So then you're able to say, restrict Peter, who's in a different unit or in different organization, from seeing these two things together. The system doesn't even show it at all and that policing is what makes business leaders actually say wow, that in itself is a huge business concern that we didn't actually always find tune and tool in a way that we ensure that it happens all the time.
Suzanne El-Moursi:This is an example of where you apply agentic AI and it's actually much better results than human beings.
Peter:Because it is a common problem. We see One of the other pieces and you were sort of touching on it there too is that fragmentation, which happens also in large organizations across data teams, where each of those data functions exists within its own silo with its own VP, and so then they don't talk across each other. So trying to move the overall data strategy forward becomes very difficult because there isn't one.
Suzanne El-Moursi:Yes, yes, Energy companies, our energy sector, and we're going into it more. They're also really old, like the utilities, and they haven't invested and they know that. They're saying we're actually making infrastructure as investments today, but that's about over the next five to seven years. I can't wait to unlock my insights when my infrastructure because they also, you know, grew through M&A themselves local utilities that get bought up by the bigger utility all over the US, different states, different regulators, regulations as well and so they often will come to us and say how can I activate this layer which is data, while I am making some of these infrastructure, but they won't be in time for me to figure out, for example, my pricing strategy when there's you know, it's a very regulated industry and what should I be doing? And so we also see that in the like, really legacy industry too, it gets overwhelming between all the different layers of the cake. As I said, from the infrastructure to the tooling, to the people, to the fragmentation, the fragmentation. To me, I just feel like we are exploring the moon and Mars and we have EVs and we still don't know. I can't get these things to come together because my last name, el Morsi, has a hyphen. I have it in this system. I don't have it in that system.
Suzanne El-Moursi:We see this a lot in our higher education universities. One of our client customers wants to do a 360 view of the student. Oh my God, your financial aid data is different from the grades that you got from basically your wellness. You know. Students there's all kinds of services are offered to students. There is no singular way and they're all great SaaS right. And then the content of in Blackboard for the courses that you took. Now, why does that matter to the university? Because everybody's trying to activate their alumni community, right, but how do you activate them when they became an alumni, when you did such a poor job knowing their student experience and have a 360 view of the student? So our very first higher education account that came on a few years ago had 300 different areas where they were pulling data from. If you were athletics and you played the sports. So just kind of, how did you come into the university? What was your performance? What happened when you threw out? It's so much nuance and opportunity in the fragmentation problem.
Peter:Yeah, I'd agree, I'm somewhat familiar with that one. There's a lot of places that data can come from and try. I was actually one of the things I was going to ask next around the if you're bringing all that data in and standardizing it to a common model so you can start to draw insights off. It is obviously a key aspect of that. So it's the ETL type, loads or the variants of that, say, depending on what order you're going to look at doing that into, actually start to standardize what the data looks like so you can start to bring those insights to the forefront looks like, so you can start to bring those insights to the forefront Totally.
Suzanne El-Moursi:You know this customer that I'm mentioning in the higher ed space. The very first set of data, they were 167 tables. We found 16 of them are empty. So it's like why is it on your list? Did you know that you have nothing in these tables? So just quickly you do discovery and it's like no, I actually didn't. And I was like, hey, I'm just being funny, this is not a trick question, but actually you have these tables that are empty, so let's just remove that. You're that discovery. Even what's here, what's in my sandbox.
Suzanne El-Moursi:And before I became an entrepreneur, I spent 16 years in big corporate GE, Healthcare, IBM and with IBM Global Services. Back in the day in the mid 90s, with the birth of the at sign, you know, all of our customers were hiring IBM Global Services to deploy the WWW, called the Internet Right, Because at that in the mid 90s the Internet was the biggest thing and a lot of business transformation. So I remember being in Bank of America. I remember being at State Farm, you know, 27 years ago, trying to adopt the Internet and do things like online banking. You know homeowners insurance policy, but the data is in Linux and made for him and it's terrible.
Suzanne El-Moursi:Pretty much it was better to do everything from the beginning, from a clean slate. That was a lot of investment, a lot of time. So I think about that and say, wow, there's still a lot of places where you just can't get a clean cut, you know, and then apply it to the AI and then be happy with the outcome. But remember, it is artificial intelligence, it is artificial. So you give it junk data. It continues to be artificial. Don't be upset with the bots.
Dave:Reflecting on some of the conversation we were having just before. We hit record and one of those being you introduced BrightHive as really helping organizations make lots and lots of data-driven decisions. That's often it's partly a data problem, partly a technical problem, but it's also a huge cultural challenge problem. Partly a technical problem, but it's also a huge cultural challenge because a lot of leaders, even if they say they want to see data, there's this huge kind of gut, feel, intuition, understanding or experience that they bring to the table, which often conflicts with that. How are you seeing that sort of cultural shift? How is it facilitated with the? You know Bright, hive and what you have there, but what do you see in that cultural change? Is it shifting? What's driving that change?
Suzanne El-Moursi:So, first of all, we make it, we make a great effort to educate whoever came to our website and decided to book a demo. You know why are you interested in BrightHive and they'll tell us all the things cost cutting tools, whatever. And let me tell you about BrightHive. Our vision, suzanne, sees a world where everybody in the organization is a much more knowledgeable worker and the work that they do is actually much more knowledgeable worker, and the work that they do is actually much more data-informed. In fact, I have been on every podcast arguing. I am going to start a KPI called Delight Is my job more delightful? Can we start measuring this as we're pushing AI to the workforce and the change that's happening? Because, in my opinion, if my boss and they did right Like I'm literally 47 today, so think 30 years ago like you're going to have to use this tool. That's it. That's how we're doing it here.
Suzanne El-Moursi:I took it on, I couldn't complain. I had to learn it. Usually, you know, the old systems were very difficult. The UI sucked and I did it. So to me, I feel that workers should be front and center to tell us on the delight of their jobs.
Suzanne El-Moursi:And so why does that matter? Because I want Peter to think about. His very first thing in the morning is to go into BrightHive. And what in the future? What will he see? Well, the hive, from our perspective, is a representation of his company, the culture. And if BrightHive is deployed enterprise-wide and it is your agentic layer in your firm's architecture, and this agentic layer is seven agents that work in unison to act as your data team?
Suzanne El-Moursi:If Peter is being asked to create a memo or to, within the next hour, bring back an answer, he knows he has what he needs. He has both his data assets and he has his data team in a box. And Peter can get on BrightHive and be able to point whatever he's looking for, whether it's the Google Analytics data, whether it's a Salesforce. We connect 350-plus connectors plus, take straight flat files, pdfs, excel, spreadsheets. He loads what he needs and he creates a project workspace and then works with the agents to figure out the answers that he may need and push them into a PowerPoint slide, push it into whatever he wants. That what just happened is to service Peter. These agents are not sitting out here doing something. They're not. They need to be told. Help me find an answer to this question. Help me find that you know the me find the next data strategy, whatever it is and I believe that Peter gets to say if that was a worthwhile use of his time and did it actually propel him further in his work and make his knowledge work actually be a lot more data informed.
Suzanne El-Moursi:So in the future, we want people across the organization we share this with whoever came to knock on our door to get a demo what is your future vision for your workforce as they adopt BrightHive across the enterprise?
Suzanne El-Moursi:Because that's what we empower. We empower that 97% that I mentioned to you is not part of the central data teams. We're empowering those folks, the 97% of smart people that are in procurement and customer success and in finance, who have really important tasks, but they do have a dependency on the data assets that the firm has. If we empower them with a full stack that is, an agentic layer protected in your cloud and the prompt engineering tools and tips, and they're able to unlock insights faster for the servicing of their roles and their organizations in a whole, that culture will show up in the hive, because when you dial, you know, you log into your hive and you see it. What I want to see, what I'm showing you is that every microcosm in the organization, regardless of what function or unit they're in, what they revealed as insights because you check it and you share it across the organization, and that's our hope is that the page where the hive exists is a representation of a more data, mature data, informed data driven type of workforce.
Peter:And you've just got to hope that it isn't the person who says that well, you've shown me the data, but it doesn't prove what I want to say, so I'm not going to believe it.
Suzanne El-Moursi:Yes, there will be. There will be that. There's has to be a wave of that. People are going if this all materializes, the growing pains out of this, the naysayers and nonbelievers you know they have to realize, yes, we are at a point where we are going back and analyzing and making progress on that debt. Right? That's exactly the point.
Suzanne El-Moursi:If 90% of the world's data was produced in the last 24 months, we do have a capacity issue. We do have a lot of people that are going to not today. They don't even trust the dashboards. They don't know when was the last relevant update to the data? A hundred percent, and that's going to continue. But I think that gap starts to close when we are showing, when we're showing the performance of the tools, when we're showing that you're still in control, when we're measuring your productivity by you know and delight. All of these things in the future should move us towards more conviction around what we have to work with. And if we don't, then we didn't, right? I mean, that's the bet we're making on AI. Then we didn't, but we made a bet on the Internet. And look where we are today. You can't live without your smartphone. You can't live without your Google Maps. Imagine MapQuest back in the day.
Dave:Imagine physical MapQuest back in the day. Imagine physical MapQuest. I'm remembering paper maps. When you got to Remember the paper maps, navigate that one.
Suzanne El-Moursi:Yeah, when.
Dave:I came to the.
Suzanne El-Moursi:United States from Egypt. Yeah, you have to map some paper all the time.
Dave:Can I? I know we're going to run out of time shortly and Peter's going to jump in and talk about the three takeaways in just a second. You mentioned the KPI of delighting delight in an organization. Have you taken that to the point where you know what the definition of the delight KPI is?
Suzanne El-Moursi:Not yet, but that is something that I would like to do. I am going to. My intention is to invite all of these early customers that are bringing an agentic data team in a box. That's what we are agentic data team in a box and rolling it out and showing people that they're being given autonomy to work with their data sets right there. They have them on their laptops, they have them in the cloud right and be able to encourage, share the insights that you have. Our goal is that anybody in IT and the business, or two business units, to have a different kind of conversation together, because they're meeting all the time. So I believe that as part of that measurement is that we're helping influence the conversations that are happening in the enterprise between marketing and sales, between finance and ops, between customer success and procurement, whatever. It is right, because they each have a set of data, they have a set of the story, they have a slice of the pie. Bring them together. Your data is great, my data is great. Together we're powerful. Let's create powerful BrightHive is a pathway to that Now and let's measure along the path what that does for the enterprise and the culture and the people, the people dynamics. And so to me I would define it around these things. But you also want the business leaders to have that buy-in and bring it in and define it from their own organizations.
Suzanne El-Moursi:For us here at Bright Hive we have, you know, coming up on the summer, so we're getting some really amazing MBA students for 12 weeks. These are students from University of Chicago Booth. When you look at the resume they're all coming from Bain and McKenzie. Their Ivy Leagues are Cornell and otherwise. They're from all over the world, right, and we know what happened when they went to McKinsey and Bain.
Suzanne El-Moursi:Right, usually, when I become a kind of a management consultant, you go there. You're there for three to five years, you learn all the frameworks and then you decide do you go in-house, right, go work at a big firm, or do you go get your MBA? So now we're taking these folks who are going to learn how to do GTM strategy agentically, right, and I am actually going to be measuring this with the university, with the Polsky Center and the Harry Davis we were just talking about this on Friday and I want to make a point to say we took very smart people that do the MBA route and the McKenzie route and the Bain route and they're going to come and work on a startup and do GTM very different than they've ever done. They don't even know the tools that I interviewed them on. And let's monitor that delight and that productivity from June all the way to September. You have to get myopic to that level to start the case. Yeah.
Peter:Yeah, hopefully you can. The MBA students I've seen try and make that leap often struggle when they hit the startup space because they think of things in terms of massive frameworks and in fact, they think of things in terms of having to be in a framework versus being able to think and respond to what the concept in front of them is and how to deal with a complex system versus one that's very structured and moving at a very different pace.
Suzanne El-Moursi:Absolutely. And when you look at go-to-market today, the entire stack is an AI stack in GTM. I mean, I know it because this is what I have deployed here. So, as I was interviewing, I'm asking you know like, clearly smart, clearly critical thinker, clearly got curiosity and hunger. Now let me ask you about the tools you use. It's like do you know X, do you know Y? What about this? And we have our stack is already six brands that we're using Like okay, that's the point is all of that is foreign to you and on the books, based on your CV, you're brilliant.
Suzanne El-Moursi:So come on here and you're running a GTM strategy that's completely agentic. Let's see how we make you more AI enabled. Because I heard this in November at the Insight Partners Scale Up AI Summit that they have every year and the CTO of Mayo Clinic said he gets all the resistance all the time from the physicians. And this is the Mayo Clinic and his response is a doctor with AI is going to displace a doctor without AI. It is not AI displacing the doctor, and that is what we are telling our physician population, which are, you know, some of the smartest people to get through medical school and be there. It is true everywhere. In my example, as the MBA, you know second year students. They're coming here, they're smart, they got the frameworks from McKinsey and everywhere else. You got to put it into practice for GTM right, and here's how you're going to do it, and so we hope to be publishing it in the fall as our little experiment on how we are affecting the labor force differently for a very well-respected population.
Peter:Well, as Dave was saying, I'm now going to ask us to wrap this up. So thank you very much, susan. It's been fantastic information, and we like to wrap this up with three key points for our listeners to take away with them, and so, on that note, I'll ask you, susan, what would your favorite takeaway from this conversation be for our audience?
Suzanne El-Moursi:I love your question and kind of validation on fragmentation of data. I love your question and kind of validation on fragmentation of data. It's just like the root canal that just never goes away, right? So recognize that, recognize the things we're trying to solve. That is point number one.
Suzanne El-Moursi:Number two that I like how, in the conversation we highlighted there will always be skeptics and our jobs as automation architects, if you will, if that's what we're going to be looked at, right, and all the fear I mean, in the end of the day, human beings always overblow the risk and underestimate the opportunity. It is a human thing. And recognize that that's what we're doing, right? So, as naysayers, as optimists, as realists, whatever you think you are doing, who you are, your profile, at the end of the day, there is a fine tuning and sharpening of the pencil on what you really want to see and how are we getting there? And socializing that it is not just, hey, we're here and then we're going to go to the moon tomorrow and we just don't know how we're going to get there, right? I think that's where a lot of the fear comes out, because then people are resistant, they don't know what to expect, they don't like to be, you know, surprised and there's a lot of risk. We're not risk takers and we overblow that component. I think it is it's important to recognize you're going to have naysayers organize the. You know the response. I mentioned the KPI. I want to measure and be systemic about why I'm doing that and where I'm trying to go. And, thirdly, that at the end of the day, when we do this well, that it isn't, you know we're solving all the world's problems.
Suzanne El-Moursi:I think we're essentially and I say this all the time we're allowing human beings to, for the first time, think about applying high intelligence. You know high human intelligence, that we have to bigger, more innovative ideas, which we never got to because we're so busy working at the grunt level of data. So, of course, from my perspective, we're applying agentic AI very uniquely. We are not a chatbot and every AI company is doing something. But for the application on data and data work, that is difficult and you ask anybody how they feel about their data, they will never tell you that they are joyful, right, and we apply and show that we've made a dent into that and kind of the rewards back to society and to the cultures of the firms that we serve. You start to take that skeptic to be a believer and they're seeing a path and it's not as fearful and it's not, you know, scary, and then you're bringing more people onto the journey and that is how you apply change right and kind of architected in society in that way.
Dave:Awesome, dave. I'm only going to add one comment to, or one observation as we're going through, and, suzanne, I think you've really helped articulate something which is very easy to see in the news, as you said, that fear mongering. Fear mongering is probably strong, but the fear that's generated by AI is coming here and what's going to happen?
Peter:Sure.
Dave:And what you've described is how it couldn't work in the use cases that we've talked about, in the sense that these are really accelerators and they empower people to do the interesting things.
Peter:Right.
Dave:When they're in a role where they're overloaded with lots and lots of demands on there, and so it starts opening up the possibility of interesting, new, more insights and learnings and so on that comes with that. So I think that one was just. It's almost a thread that's come through the whole thing of this. It isn't replacing my hands at the keyboard so much as making the time I spend at the keyboard more valuable, more interesting, more like generating more insights and learnings and so on.
Suzanne El-Moursi:Absolutely yeah.
Peter:Yeah, the optimism is great. I mean, I think it's the right way to look at how these tools empower us as we look forward at all of the different activities that we do in an organization. There is so much of that work that we just never have time to get to. So looking at AI as an accelerator to help us remove a lot of that grunt work to get to the interesting pieces that really help solve the big problems is definitely a massive enabler. With all of that said, I think it's been a fantastic conversation, so thank you so much for your time and I think we'll wrap it up there for today, and people can reach out to us at feedback at definitelymaybeagilecom, and it's a pleasure, as always. Thank you both.
Suzanne El-Moursi:Pleasure is mine. Thank you very much. Thanks a lot.